import pandas as pd
import matplotlib.pyplot as plt
import matplotlib
import matplotlib.font_manager as fm
import seaborn as sns
import pandas as pd
from IPython.display import display
import numpy as np
pd.options.display.max_columns = None
%matplotlib inline
plt.rcParams['font.family'] = 'NanumGothic'
matplotlib.rcParams['axes.unicode_minus'] = False
font_path = '/usr/share/fonts/truetype/nanum/NanumGothic.ttf'
font_prop = fm.FontProperties(fname=font_path).get_name()
matplotlib.rcParams['font.family'] = font_prop
sns.set_palette('Set2')
sns_color = sns.color_palette('pastel')[0:5]
regular_season = pd.read_csv('../datas/Regular_Season_Batter.csv')
regular_season_day = pd.read_csv('../datas/Regular_Season_Batter_Day_by_Day_b4.csv')
display(pd.DataFrame({'regular_season': regular_season.isna().sum(), 'regular_season_day': regular_season_day.isna().sum()}).fillna(''))
'''
앞서 각 데이터의 null 값을 모두 채웠기 때문에 없다.
첫 연봉은 제대로된 정보가 없고, 있더라도 조금씩 달라서 제외했다.
''';
|
regular_season |
regular_season_day |
2B |
0.0 |
0.0 |
3B |
0.0 |
0.0 |
AB |
0.0 |
0.0 |
BB |
0.0 |
0.0 |
CS |
0.0 |
0.0 |
E |
0.0 |
|
G |
0.0 |
|
GDP |
0.0 |
0.0 |
H |
0.0 |
0.0 |
HBP |
0.0 |
0.0 |
HR |
0.0 |
0.0 |
OBP |
0.0 |
|
OPS |
0.0 |
|
R |
0.0 |
0.0 |
RBI |
0.0 |
0.0 |
SB |
0.0 |
0.0 |
SLG |
0.0 |
|
SO |
0.0 |
0.0 |
TB |
0.0 |
|
avg |
0.0 |
|
avg1 |
|
0.0 |
avg2 |
|
0.0 |
batter_id |
0.0 |
0.0 |
batter_name |
0.0 |
0.0 |
career |
0.0 |
|
country |
0.0 |
|
date |
|
0.0 |
hand |
0.0 |
|
height/weight |
0.0 |
|
opposing_team |
|
0.0 |
pos |
0.0 |
|
position |
0.0 |
|
starting_salary |
1067.0 |
|
team |
0.0 |
|
year |
0.0 |
0.0 |
year_born |
0.0 |
|
'''
입력된 데이터 중에서 잘못 입력된 데이터들이 있다.
그 데이터들을 수정했다.
수정하기 전에 각 컬럼이 어떤 것인지 다시 한 번 확인해 보았다.
''';
컬럼 이름 | 뜻 | 컬럼 이름 | 뜻 |
---|
batter_id | 타자 ID (고유 식별자) | HBP | 몸에 맞는 공 (Hit by Pitch) |
batter_name | 타자 이름 | SO | 삼진 (Strikeouts) |
year | 시즌 연도 | GDP | 병살타 (Grounded Into Double Play) |
team | 소속 팀 이름 | SLG | 장타율 (Slugging Percentage, 총 루타 / 타수) |
avg | 타율 (Batting Average, 안타 수 / 타수) | OBP | 출루율 (On-base Percentage, 출루 횟수 / 타석) |
G | 경기 수 (Games Played) | E | 실책 수 (Errors) |
AB | 타수 (At-Bats) | height/weight | 신장/체중 (Height/Weight) |
R | 득점 (Runs Scored) | year_born | 출생 연도 (Year Born) |
H | 안타 수 (Hits) | position | 포지션 (Position) |
2B | 2루타 (Doubles) | career | 경력 (Career, 몇 년 차인지) |
3B | 3루타 (Triples) | starting_salary | 연봉 (Starting Salary) |
HR | 홈런 수 (Home Runs) | OPS | OPS (출루율 + 장타율, On-base Plus Slugging) |
TB | 루타 수 (Total Bases) | pos | 세부 포지션 (Position Detail, 예: IF, OF 등) |
RBI | 타점 (Runs Batted In) | hand | 타격/투구 손잡이 (Hand, 예: R-우타, L-좌타) |
SB | 도루 (Stolen Bases) | country | 국적 (Country) |
CS | 도루 실패 (Caught Stealing) | | |
BB | 볼넷 (Base on Balls) | | |
'''
먼저 기존에 잘못 계산했기 때문에 잘못된 값이 들어가 있다.
그래서 값을 먼저 처음 값으로 되돌리고 진행한다.
'''
regular_season['SLG'] = pd.read_csv('../datas/62540_KBO_prediction_data/Regular_Season_Batter.csv', encoding='utf-8')['SLG']
regular_season['OBP'] = pd.read_csv('../datas/62540_KBO_prediction_data/Regular_Season_Batter.csv', encoding='utf-8')['OBP']
display(regular_season[(regular_season['SLG'] == 0) & (regular_season['H'] > 0)].iloc[:, :22].head(3))
'''
SLG = (단타 + 2루타 *2 + 3루타 * 3 + 홈런 * 4) / 타수
SLG = H + (2B * 2) + (3B * 3) + (HR * 4) / AB
''';
regular_season['SLG'] = regular_season.apply(lambda x: round(sum([x['H'], x['2B'] * 2, x['3B'] * 3, x['HR'] * 4]) / x['AB'], 3) if x['AB'] != 0 else 0, axis=1)
pd.concat(
[regular_season[['H', '2B', '3B', 'HR', 'AB']].map(int),
pd.read_csv('../datas/62540_KBO_prediction_data/Regular_Season_Batter.csv', encoding='utf-8')['SLG'].reset_index(drop=True),
regular_season['SLG'].reset_index(drop=True)],
axis=1
)
|
batter_id |
batter_name |
year |
team |
avg |
G |
AB |
R |
H |
2B |
3B |
HR |
TB |
RBI |
SB |
CS |
BB |
HBP |
SO |
GDP |
SLG |
OBP |
478 |
62 |
김주찬 |
2000 |
삼성 |
0.313 |
60 |
48 |
22 |
15 |
3 |
2 |
0 |
22 |
5 |
7 |
2 |
3 |
1 |
16 |
0 |
0.0 |
0.0 |
746 |
109 |
박기혁 |
2000 |
롯데 |
0.333 |
5 |
3 |
1 |
1 |
0 |
0 |
0 |
1 |
1 |
0 |
0 |
0 |
0 |
2 |
0 |
0.0 |
0.0 |
1457 |
207 |
이범호 |
2000 |
한화 |
0.162 |
69 |
74 |
11 |
12 |
7 |
0 |
1 |
22 |
3 |
1 |
0 |
10 |
1 |
21 |
2 |
0.0 |
0.0 |
|
H |
2B |
3B |
HR |
AB |
SLG |
SLG |
0 |
62 |
9 |
0 |
8 |
183 |
0.519 |
0.612 |
1 |
0 |
0 |
0 |
0 |
1 |
0.000 |
0.000 |
2 |
19 |
2 |
3 |
1 |
86 |
0.349 |
0.419 |
3 |
80 |
7 |
4 |
2 |
311 |
0.325 |
0.367 |
4 |
16 |
3 |
2 |
1 |
101 |
0.257 |
0.317 |
... |
... |
... |
... |
... |
... |
... |
... |
2449 |
0 |
0 |
0 |
0 |
5 |
0.000 |
0.000 |
2450 |
0 |
0 |
0 |
0 |
2 |
0.000 |
0.000 |
2451 |
0 |
0 |
0 |
0 |
10 |
0.000 |
0.000 |
2452 |
34 |
6 |
2 |
1 |
117 |
0.402 |
0.479 |
2453 |
4 |
1 |
0 |
1 |
24 |
0.333 |
0.417 |
2454 rows × 7 columns
'''
OBP = (안타+볼넷+몸에 맞은 공)÷(타수+볼넷+몸에 맞은 공+희생플라이)
OBP = (H + BB + HBP) / (AB + BB + HBP + "") 이 데이터에는 희생플라이 컬럼이 없다.
그래서 문제가 있는 행은 제거한다.
'''
drop_index = regular_season.loc[
(sum([regular_season['H'] > 0, regular_season['BB'] > 0, regular_season['HBP']]) > 0) & (regular_season['OBP'] == 0) |
(sum([regular_season['AB'] > 0, regular_season['BB'] > 0, regular_season['HBP']]) > 0) & (regular_season['OBP'] == 0)
].index
regular_season = regular_season.drop(drop_index).reset_index(drop=True)
regular_season.head(2)
|
batter_id |
batter_name |
year |
team |
avg |
G |
AB |
R |
H |
2B |
3B |
HR |
TB |
RBI |
SB |
CS |
BB |
HBP |
SO |
GDP |
SLG |
OBP |
E |
height/weight |
year_born |
position |
career |
starting_salary |
OPS |
pos |
hand |
country |
0 |
0 |
가르시아 |
2018 |
LG |
0.339 |
50 |
183 |
27 |
62 |
9 |
0 |
8 |
95 |
34 |
5 |
0 |
9 |
8 |
25 |
3 |
0.612 |
0.383 |
9 |
177cm/93kg |
1985년 04월 12일 |
내야수(우투우타) |
쿠바 Ciego de Avila Maximo Gomez Baez(대) |
NaN |
0.902 |
내야수 |
우타 |
외국인 |
1 |
1 |
강경학 |
2014 |
한화 |
0.221 |
41 |
86 |
11 |
19 |
2 |
3 |
1 |
30 |
7 |
0 |
0 |
13 |
2 |
28 |
1 |
0.419 |
0.337 |
6 |
180cm/72kg |
1992년 08월 11일 |
내야수(우투좌타) |
광주대성초-광주동성중-광주동성고 |
10000만원 |
0.686 |
내야수 |
좌타 |
한국인 |
plt.figure(figsize=(10, 5))
plt.plot('AB', 'OPS', data=regular_season, marker='o', linestyle='none', markersize=2, color='green', alpha=0.4)
plt.xlabel('AB(타수)', fontsize=14)
plt.ylabel('OPS(장타율)', fontsize=14)
plt.vlines(30, ymin=min(regular_season['OPS']), ymax=max(regular_season['OPS']), linestyles='dashed', colors='red')
plt.show()
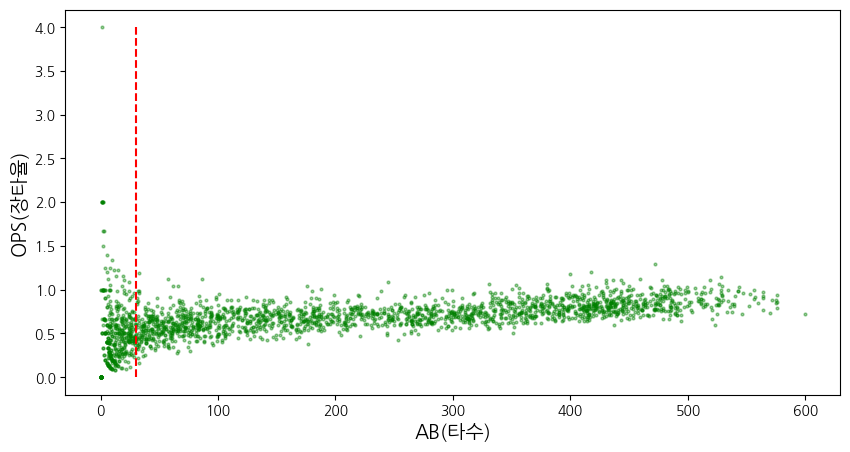
Q1 = regular_season['OPS'].quantile(0.25)
Q3 = regular_season['OPS'].quantile(0.75)
IQR = Q3 - Q1
regular_season.loc[
(regular_season['OPS'] < (Q1 - 1.5 * IQR))|
(regular_season['OPS'] > (Q3 + 1.5 * IQR))
].sort_values(by=['AB'], axis=0, ascending=False)[['batter_name', 'AB', 'year', 'OPS']].head(10)
|
batter_name |
AB |
year |
OPS |
748 |
박병호 |
528 |
2015 |
1.150000 |
2212 |
테임즈 |
472 |
2015 |
1.293656 |
87 |
강정호 |
418 |
2014 |
1.200156 |
749 |
박병호 |
400 |
2018 |
1.175000 |
1253 |
유재신 |
33 |
2018 |
1.192000 |
2234 |
한승택 |
33 |
2013 |
0.165000 |
2046 |
채상병 |
32 |
2002 |
0.215909 |
391 |
김원섭 |
25 |
2005 |
0.116923 |
573 |
나주환 |
23 |
2013 |
0.174000 |
1469 |
이여상 |
22 |
2013 |
0.090909 |
major_ticks = list(np.round(np.linspace(7.01, 7.31, 31), 2))
july = (regular_season_day['date'] >= 7) & (regular_season_day['date'] < 8)
plt.plot(major_ticks, regular_season_day['date'].loc[july].value_counts().sort_index(), marker='o')
plt.xticks(major_ticks, rotation=90)
plt.show()
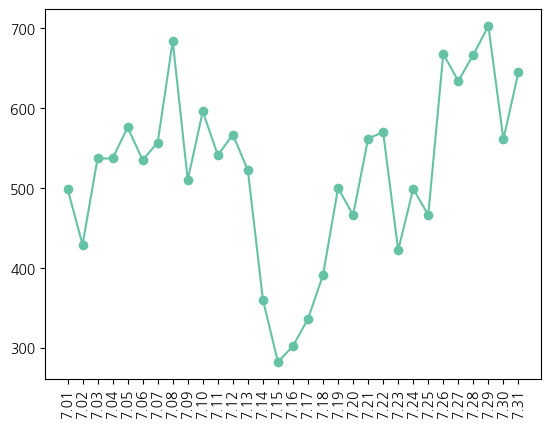