[논문리뷰] UNet++: A Nested U-Net Architecturre for Medical Image Segmentation
Abstract
- UNet 구조보다 좀 더 nested된 형태의 구조를 제안 → 인코더와 디코더 특성 맵의 semantic gap을 줄이기 위해 고안
Motivation
- 다음 가정을 기반으로 더 높은 정확도를 가지는 semantic segmantation 모델을 구성하고자 함
- 가정: 고해상도의 encoder network의 특성 맵이 decoder network에서 특성 맵과 합쳐지기 전에, 풍부한 표현을 가질 수 있도록 구성 → decoder network와 encoder network의 semantic gap을 줄임으로써 학습을 더 용이하게 할 수 있음 → 디테일들을 더 잘 포착할 수 있도록 유도
구조 및 특성
구조
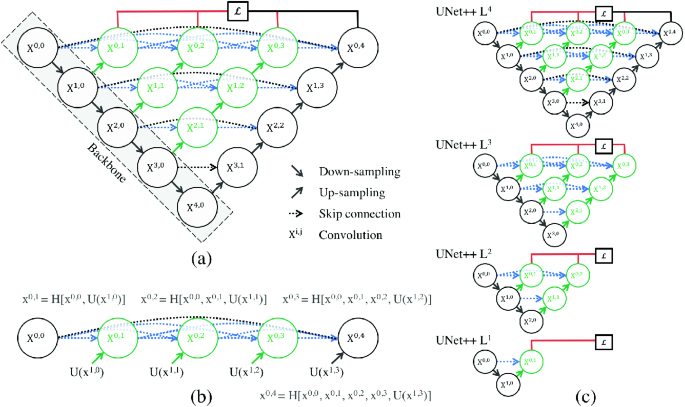
특성
- UNet 구조와 비교하여 다른 점
- (초록색) convolutional layers on skip pathway
- (파란색) dense skip connection
→ down-sample 결과를 다시 up-sample한 결과를 붙임으로써 decoder network와 encoder network 사이의 semantic gap을 줄이고자 함
- (빨간색) deep supervision: 모델이 다음 두 가지 모드로 동작할 수 있도록 제안
- accurate mode: 모든 segmenation branch들의 출력의 평균을 취할 때 동작하는 모드
- fast mode: segmentation branch에서 최종 segmentation 결과를 선택할 때 동작하는 모드 (모델의 복잡도를 결정)
- loss function = binary cross-entropy loss + dice coefficient
L(Y,Y^)=−N1Σb=1N(21⋅Yb⋅logY^b+Yb+Yb^2⋅Yb⋅Yb^)
한계점
- 복잡한 connection으로 인해 파라미터의 수가 많고, 메모리를 많이 사용
- full-scale에서 정보를 충분히 탐색하지 못하므로 정확도가 다소 떨어짐