Clark, K., Luong, M. T., Le, Q. V., & Manning, C. D. (2020). Electra: Pre-training text encoders as discriminators rather than generators. arXiv preprint arXiv:2003.10555.
1. Introduction
ELECTRA(Efficiently Learning an Encoder that Classifies Token Replacements Accurately)는 2020년 구글 리서치 팀에서 발표한 언어모델입니다. 기존의 BERT를 비롯하여 많은 언어모델들이 사용했던 pre-training 기법인 Masked Language Model(MLM)은 입력토큰의 일부를 마스크 토큰([mask])으로 치환하고 원래 토큰으로 예측하는 방식으로 학습을 진행하였습니다. 하지만 이 기법은 전체 토큰 중 일부(BERT의 경우 15%)만 학습시킬 수 있어 입력에 대해 학습하는 데이터의 양이 적기 때문에, 학습을 위해 필요한 컴퓨팅 비용이 많이 든다는 문제가 있었습니다. ELECTRA는 새로운 pre-training 기법을 제안하여 이 문제를 해결하였습니다.
2. Method
ELECTRA는 BERT와는 다른 새로운 pre-training 기법으로 대체 토큰 탐지(RTD, replaced token detection)를 제시하였습니다. RTD는 Transformer 양방향 인코더 구조를 가지는 Generator와 Discriminator 두개의 네트워크로 구성되어 있습니다.
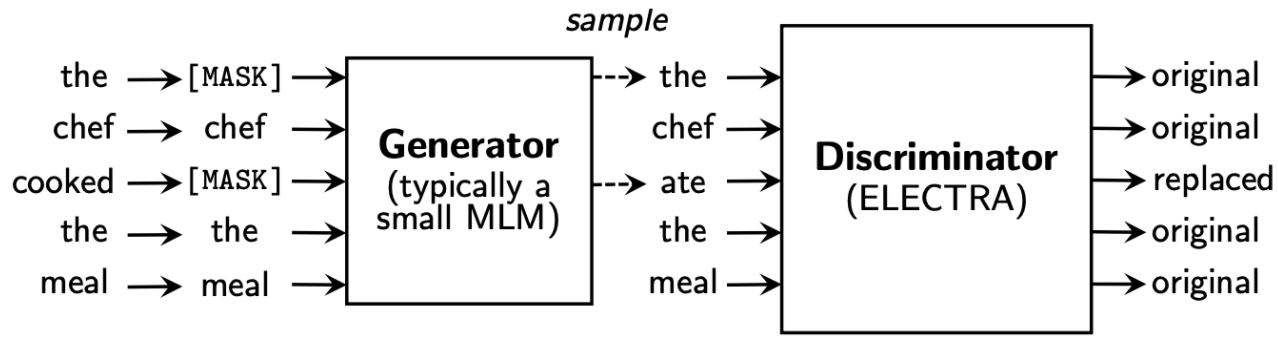
- Generator는 기존의 BERT의 MLM 학습 방법과 동일한 매커니즘을 가집니다. 입력 x=[x1,x2,…,xn]에 대해 마스킹할 위치의 집합 m=[m1,m2,…,mk]을 결정하고 결정된 토큰을 [MASK]로 치환합니다.
xmasked=REPLACE(x,m,[MASK]) xmasked에 대해 Generator에서 원래의 토큰이 무엇인지 예측하고, 일부 토큰을 가짜 정보로 대체(xcorrupt)합니다. 위 그림에서는 x = [the, chef, cooked, the, meal]에서 ‘cooked’이라는 원래정보가 ‘ate’으로 대체된 것을 확인하실 수 있습니다. 이를 수식으로 아래과 같이 표현할 수 있습니다.pG(xt∣x)=exp(e(xt)ThG(x)t)/x′∑exp(e(x′)ThG(x)t) x자리에 xmasked가 주어졌을 때 토큰의 위치 xt의 분포는 Generator를 통과한 Softmax분포로 표현됩니다. 이를 바탕으로 가장 높은 확률 값을 가지는 토큰을 샘플링하여 대체 토큰 시퀀스로 치환(xcorrupt)할 수 있습니다.xcorrupt =REPLACE(x,m,x^)x^∼pG(xi∣xmasked ) for i∈m
- Discriminator는 두 개의 Transformer encoder로 이루어져 있습니다. Generator가 생성한 가짜 정보가 포함된 토큰 시퀀스를 입력으로 하고, 모든 입력 토큰에 대해 각 토큰이 실제인지 아닌지를 구별(이진 분류, binary classfication)해줍니다. 수식은 아래와 같습니다.
D(xcorrupt ,t)=sigmoid(wThD(xcorrupt )t)
3. Result
ELECTRA는 Masked Language Model(MLM)보다 빠르고 효과적으로 학습하여 학습의 효율을 높였습니다. 아래 그래프는는 동일한 성능을 내기위해서 ELECTRA의 RTD가 MLM에 비해 더 적은 컴퓨팅 비용을 필요로 한다는 것을 보여줍니다.
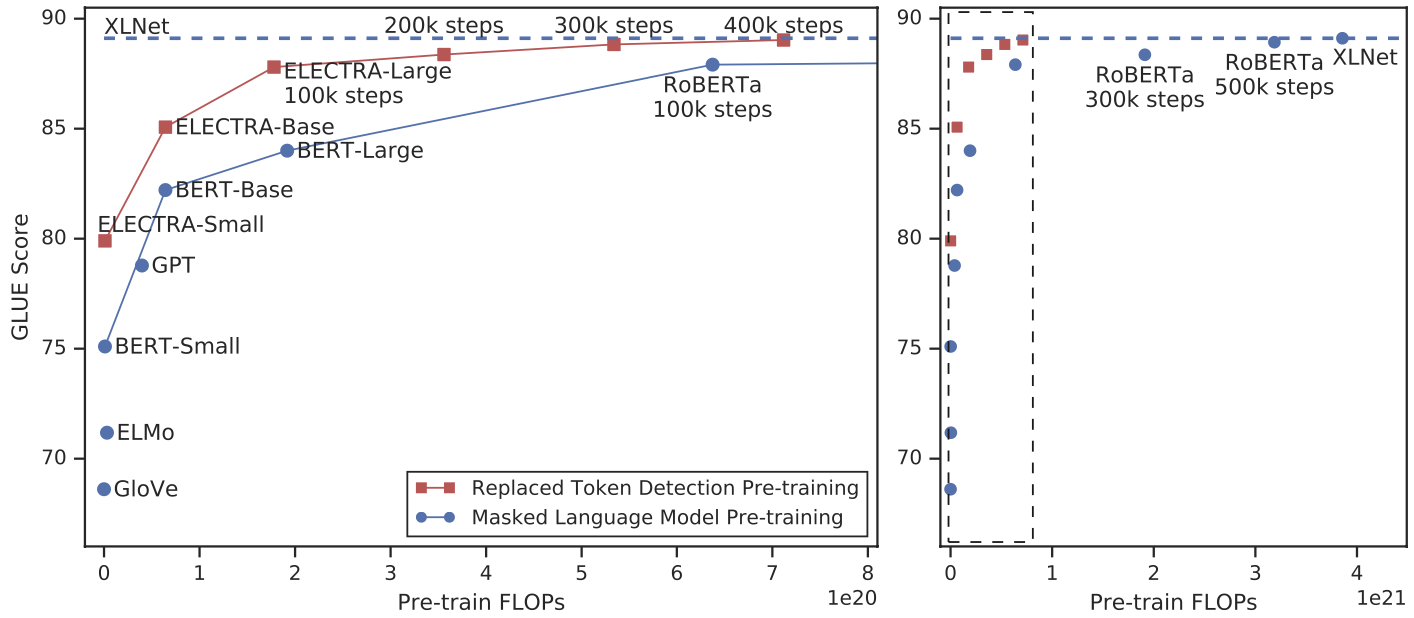
이러한 결과가 나올 수 있는 것은 MLM은 입력의 15%정도만 학습할 수 있는 반면, RTD의 경우 모든 입력 토큰에 대해서 학습할 수 있다는 점에 있습니다.
Small 모델에서 GPU 하나로 4일간 학습한 모델이 계산량(FLOPs)이 30배 가량인 GPT를 능가하였고, Large 모델에서도 RoBERTa나 XLNet 대비 1/4의 계산량(FLOPs)으로 비슷하거나 향상된 성능을 보여주었습니다.