Mathematical reasoning benchmark in Visaul Contexts
INTRODUCTION
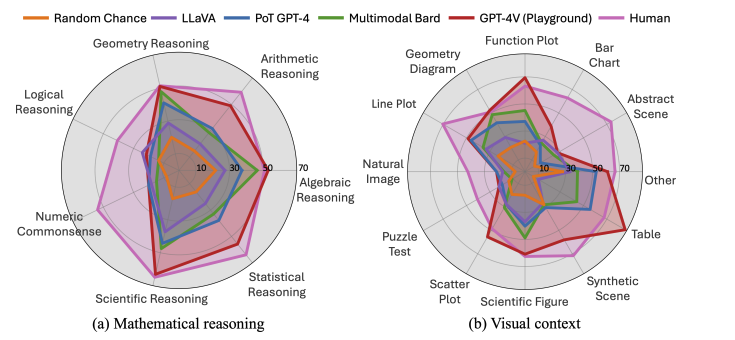
- Mathematical reasoning은 logical thinking, domain-specific knowledge, multistep reasoning이 필요한 task
- 이 복잡성은 textual 뿐만 아니라, visual에도 동등하게 요구됨
- ChartQA 등이 vision-language setting에서 mathematical reasoning에 적합하게 생성된 데이터셋이나 geometry, bar charts와 같은 specific task에 의존
- general한 VQA등이 있으나, 이는 mathematical reasoning에 필요한 질문의 일부만 포함되어 있음
- LLM과 LMM은 눈부신 발전을 하였으나, 이를 systemic하게 조사한 자료는 아직 존재하지 않음
- 따라서 visually intensive한 mathematical reasoning system을 개발하고, LLM과 LMM의 rigorous reasoning task의 발전을 평가하여야 함
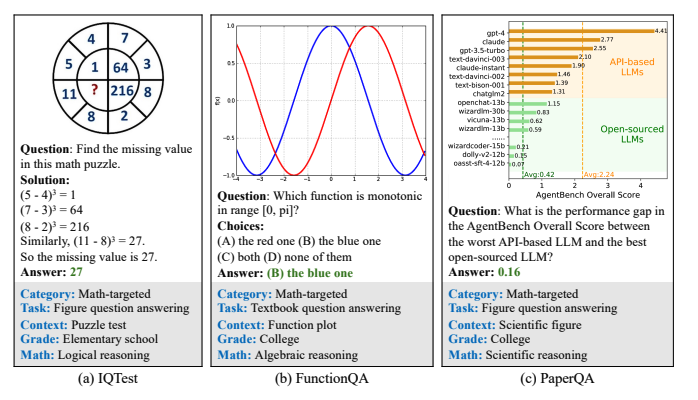
- 우리는 mathematical reasoning task를 재정립하고, IQTest, FunctionQA, PaperQA 등의 새로운 데이터셋을 추가함
- 또한 12개의 foundation model을 통해 이를 다양한 세팅으로 (zero-shot, few-shot, CoT) 평가하여 human performance와 비교함
- GPT-4의 latest version인 GPT-4V가 SOTA임을 확인
THE MATHVISTA DATASET
Collection Guidelines
- 이전 mathmatical reasoning dataset은 visual을 간과한다는 한계가 존재
- 우리는 이러한 간극을 매우고자 MathVista dataset을 구성
- real-world application을 미러링
- 더 나은 평가를 위해 visual context와 mathematical skill을 incorporate
- 다양한 수준의 challenge와 current model의 potential limiation을 uncover
- 결정론적인 비교에 강건한 평가지수를 제시
- 우리는 5가지 primary task에 집중함
- FQA(figure question answering) - 다수의 차트와 plot들에 대해 statistical reasoning
- GPS(geometry problem solving) - geometrical topic에 대한 task
- MWP(math word problem) - 평범한 상황에서의 arithmetic reasoning
- TQA(textbook question answering) - scientific topic과 figure에 대해 knowledge-intensive reasoning
- VQA(visual question answering)